Python
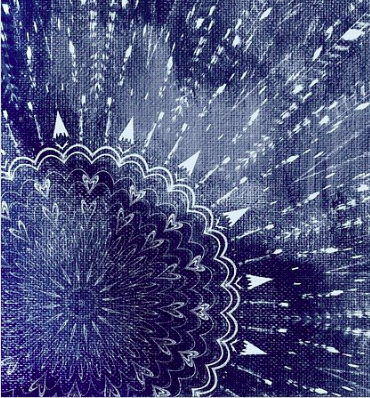
PyTorch Tensors
This blog post contains following topics related to tensors:
- Import
- Version
- Declarations
- item()
- Zero, identity and linspace
- Random generator
- Datatypes
- Operation with constant
- Element wise operations
- Matrix multiplication
- Matrix inversion
- Transpose of matrix
- Determinant of matrix
- Indexing and slicing
- Broadcasting
- Reshape
- Squeeze and unsqueeze
- Concatenate and stack
- Reorganize the data element
- numpy and pytorch tensor
- Operations on complex numbers
Import
#import the library
import torch
Version
torch.__version__
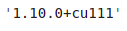
Declaration of Tensors
#Basic 1-dimension Tensor
x = torch.Tensor([5])
print('The type of x is: ',type(x))
print('The size of x is: ',x.size())
print('The dimension of x is: ',x.ndim)

#Basic 3-dimension Tensor with only one element
x = torch.Tensor([[[5]]])
print('The type of x is: ',type(x))
print('The size of x is: ',x.size())
print('The dimension of x is: ',x.ndim)

#x is a 3-d tensor
#y is a 2-d tensor
#z is a 1-d tensor
x = torch.Tensor([[[5,2],[5,2]],
[[1,2],[2,3]]
])
y = torch.Tensor([[6,2],[8,9]])
z = torch.Tensor([5,8])
print(f'The dimension of x is {x.ndim} and size of x is {x.size()}\n')
print(f'The dimension of y is {y.ndim} and size of x is {y.size()}\n')
print(f'The dimension of z is {z.ndim} and size of z is {z.size()}\n')
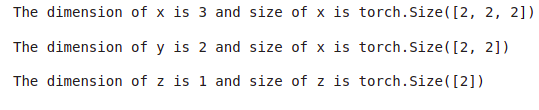
Item()
x = torch.Tensor([5])
print(x.item())
print(type(x.item()))
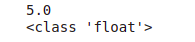
x = torch.Tensor([[5,5,5,5]])
print(x.item())
print(type(x.item()))
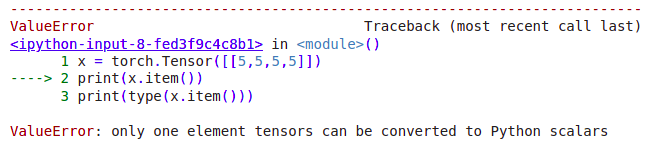
x = torch.Tensor([[5,5,5,5]])
print(x[0][0].item())
print(type(x[0][0].item()))
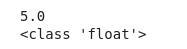
Zero, identity and linspace
#Zero
v = torch.zeros(3,3)
print(v)
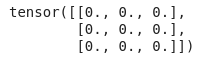
# Create an identity 2x2 tensor
I = torch.eye(2,2)
print(I)
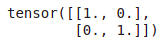
#ones
x =torch.ones(3,3)
print(x)
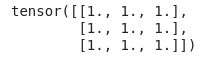
x = torch.ones_like(I)
print(x)
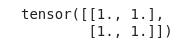
x = torch.linspace(1,100,10)
print(x)

#Initialize Tensor with a range of value
v = torch.arange(5)
print(v)
v = torch.arange(0, 5, step=1)
print(v)
v = torch.arange(0, 5, step=0.5)
print(v)

#log scale Tensor
# Size 5: 1.0e-10 1.0e-05 1.0e+00, 1.0e+05, 1.0e+10
v = torch.logspace(start=-10, end=10, steps=5)
print(v)

#Initialize a ByteTensor
c = torch.ByteTensor([0, 1, 1, 0])
print(c)

Random generator
#uniform distribution between 0 and 1
x = torch.rand(4)
print(x)

x = torch.rand(2,2)
print(x)

x = torch.rand(2,2,3)
print(x)
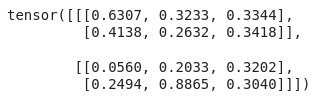
#torch.randint(min,max,(size))
x = torch.randint(1,6,(2,2))
print(x)
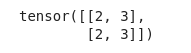
#This function returns a tensor with a random number from a standard normal distribution
#with a mean 0 and a variance of 1.
x = torch.randn((2,2))
print(x)
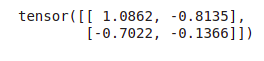
#returns a tensor of random numbers from a separate normal distribution
#whose mean and standard deviation are given.
x = torch.normal(2,3,(3,3))
print(x)

random_seed = 1
torch.manual_seed(random_seed)
print(torch.rand(1,3))
torch.rand(1)

#random permutation of integers from 0 to 3 without seed
v = torch.randperm(10)
print(v)
#We get different output everytime

#random permutation of integers from 0 to 3 with seed
random_seed = 1
torch.manual_seed(random_seed)
v = torch.randperm(10)
print(v)
#We get the same output

Different datatypes
# create a tensor with unsigned integer type of 8 bits size
a = torch.tensor([100, 200, 2, 3, 4], dtype=torch.uint8)
# display tensor
print(a)
# display data type
print(a.dtype)

# create a tensor with signed integer type of 8 bits size
a = torch.tensor([100, 200, 2, 3, 4], dtype=torch.int8)
# display tensor
print(a)

# create a tensor with signed integer type of 16 bits size
a = torch.tensor([100, 200, 200000, 3, 4], dtype=torch.int16)
# display tensor
print(a)

# create a tensor with signed integer type of 32 bits size
a = torch.tensor([100, 200, 200000, 3, 4], dtype=torch.int32)
# display tensor
print(a)

# create a tensor with floating point type of 32 bits size
a = torch.tensor([100, 200, 200000, 3, 4], dtype=torch.float)
# display tensor
print(a)

# create a tensor with floating point type of 64 bits size
a = torch.tensor([100, 200, 200000, 3, 4], dtype=torch.float64)
# display tensor
print(a)

# create a tensor with floating point type of 64 bits size or double
a = torch.tensor([100, 200, 200000, 3, 4], dtype=torch.double)
# display tensor
print(a)

# create a complex tensor
a = torch.tensor([1+2j,2+3j], dtype=torch.cfloat)
#display tensor
print(a)
# display data type
print(a.dtype)
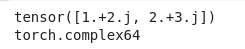
# create a tensor with bool type
a = torch.tensor([100, 200, 2, 3, 4], dtype=torch.bool)
# display tensor
print(a)
# display data type
print(a.dtype)

# create a tensor with bool type
a = torch.tensor([[0, 1],
[1,0]], dtype=torch.bool)
# display tensor
print(a)
# display data type
print(a.dtype)
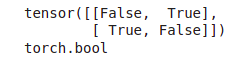
Operations with constant
x = torch.Tensor([5])
y = torch.Tensor([[5,5],
[1,1]])
print('Multiplication with constant: \n')
print(5*x)
print(5*y)
print('\naddition with a constant: \n')
print(5+x)
print(5+y)
print('\ndivision with a constant: \n')
print(x/5)
print(y/5)
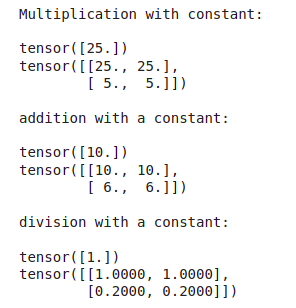
Element wise operations
x = torch.Tensor([5,6])
y = torch.Tensor([1,2])
#Addition
print('Addition: ',x+y)
print('Subtraction: ',x-y)
print('Multiplication: ',x*y)
print('Division: ',x/y)
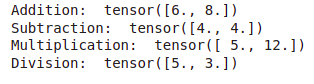
a = torch.tensor([[0, 1],
[1,0]], dtype=torch.bool)
b = torch.tensor([[0, 1],
[1,1]], dtype=torch.bool)
#Bitwise Or
print(f'Result of bitwise or:\n {torch.bitwise_or(a,b)}\n')
#Bitwise And
print(f'Result of bitwise and:\n {torch.bitwise_and(a,b)}\n')
#Bitwise Xor
print(f'Result of bitwise xor:\n {torch.bitwise_xor(a,b)}\n')
#Bitwise Not
print(f'Result of bitwise not:\n {torch.bitwise_not(a)}\n')
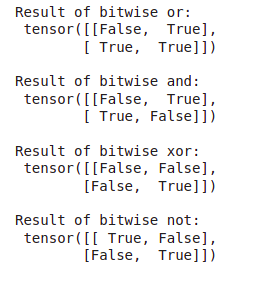
#shift operations
a = torch.tensor([[4]
])
#Bitwise left shift
print(f'Result of bitwise_left_shift:\n {torch.bitwise_left_shift(a,1)}\n')
#Bitwise right shift
print(f'Result of bitwise_right_shift:\n {torch.bitwise_right_shift(a,1)}\n')
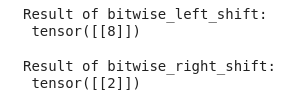
Matrix multiplication
a = torch.Tensor([5,2])
b = torch.Tensor([2,3])
c = torch.matmul(a,b)
print(c)
print(c.dtype)
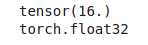
a = torch.Tensor([[5,2]])
b = torch.Tensor([2,3])
print('The size of a is: ',a.size())
print('The size of b is: ',b.size())
c = torch.matmul(a,b)
print('The size of c is: ',c.size())
print('The result of matrix multiplication is: ',c)
print('The data type of c is',c.dtype)
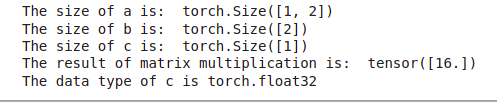
a = torch.Tensor([[5,2]])
b = torch.Tensor([[2,3]])
print('The size of a is: ',a.size())
print('The size of b is: ',b.size())
c = torch.matmul(a,b)
print('The size of c is: ',c.size())
print('The result of matrix multiplication is: ',c)
print('The data type of c is',c.dtype)
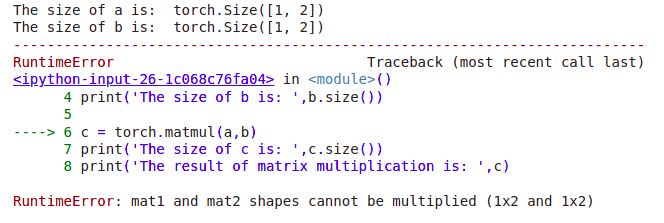
a = torch.Tensor([[5,2]])
b = torch.Tensor([[2],
[3]])
print('The size of a is: ',a.size())
print('The size of b is: ',b.size())
c = torch.matmul(a,b)
print('The size of c is: ',c.size())
print('The result of matrix multiplication is: ',c)
print('The data type of c is',c.dtype)

a = torch.Tensor([[5,2]])
b = torch.Tensor([[2,2],
[3,3]])
print('The size of a is: ',a.size())
print('The size of b is: ',b.size())
c = torch.matmul(a,b)
print('The size of c is: ',c.size())
print('The result of matrix multiplication is: ',c)
print('The data type of c is',c.dtype)

a = torch.Tensor([[[5,2],
[1,2]],
[[5,2],
[1,2]]
])
b = torch.Tensor([[[2,2],
[3,3]]])
print('The size of a is: ',a.size())
print('The size of b is: ',b.size())
c = torch.matmul(a,b)
print('The size of c is: ',c.size())
print('The result of matrix multiplication is: \n',c)
print('The data type of c is',c.dtype)
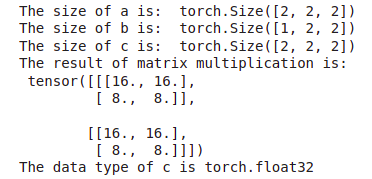
Matrix inversion
x = torch.Tensor([[5]])
print('The type of x is: ',type(x))
print('The size of x is: ',x.size())
print('The dimension of x is: ',x.ndim)
print('\n')
y = torch.inverse(x)
print('The inversion of x is y: ',y)
torch.matmul(x,y)
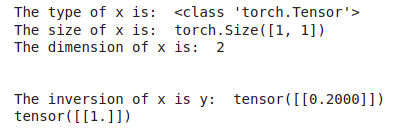
x = torch.Tensor([[5,2]])
print('The type of x is: ',type(x))
print('The size of x is: ',x.size())
print('The dimension of x is: ',x.ndim)
print('\n')
y = torch.inverse(x)
print('The inversion of x is y: ',y)
torch.matmul(x,y)
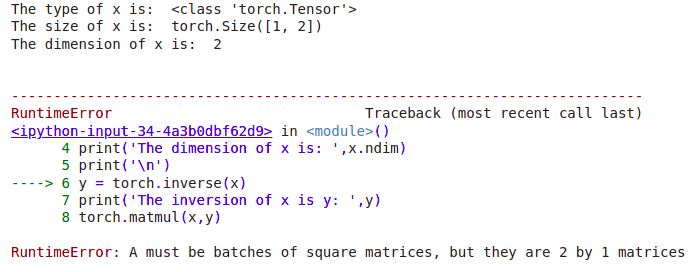
x = torch.Tensor([[5,2],
[2,5]])
print('The type of x is: ',type(x))
print('The size of x is: ',x.size())
print('The dimension of x is: ',x.ndim)
print('\n')
y = torch.inverse(x)
print('The inversion of x is y: \n',y,'\n')
torch.matmul(x,y)
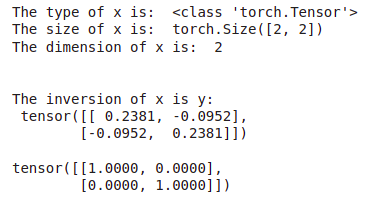
x = torch.Tensor([[[5,2],
[2,5]]])
print('The type of x is: ',type(x))
print('The size of x is: ',x.size())
print('The dimension of x is: ',x.ndim)
print('\n')
y = torch.inverse(x)
print('The inversion of x is y: \n',y,'\n')
torch.matmul(x,y)
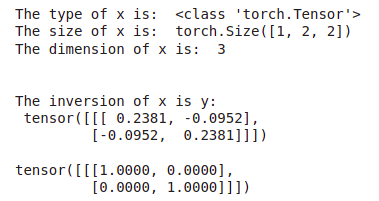
x = torch.Tensor([
[[5,2],
[2,5]],
[[5,2],
[2,5]]
])
print('The type of x is: ',type(x))
print('The size of x is: ',x.size())
print('The dimension of x is: ',x.ndim)
print('\n')
y = torch.inverse(x)
print('The inversion of x is y: \n',y,'\n')
torch.matmul(x,y)
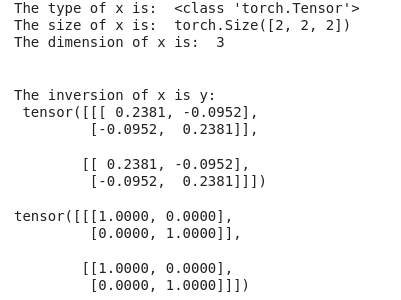
x = torch.Tensor([
[[5,2,1],
[2,5,2],
[1,1,1]],
[[5,2,1],
[2,5,5],
[1,2,3]]
])
print('The type of x is: ',type(x))
print('The size of x is: ',x.size())
print('The dimension of x is: ',x.ndim)
print('\n')
y = torch.inverse(x)
print('The inversion of x is y: \n',y,'\n')
torch.matmul(x,y)
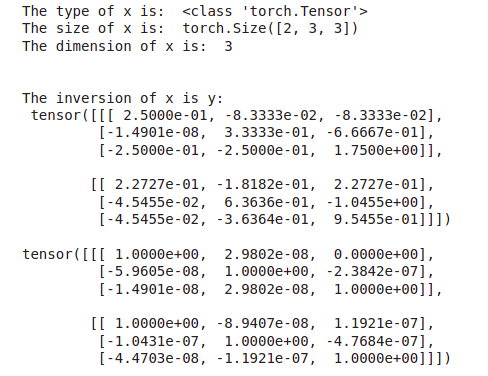
Transpose of matrix
x = torch.randn(2, 3)
print(x)
print('\nThe transpose of matrix: \n',torch.transpose(x,0,1))
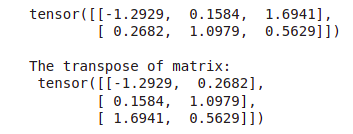
x = torch.randn(2, 3,2)
print(x)
print('\nThe transpose of matrix: \n',torch.transpose(x, 0, 1))
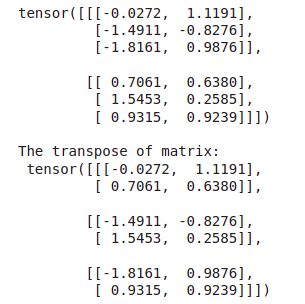
x = torch.randn(2, 3,2)
print(x)
print('\nThe transpose of matrix: \n',torch.transpose(x, 0,2))
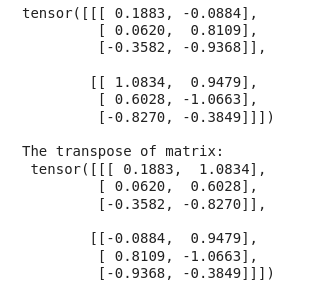
Determinant of matrix
x = torch.Tensor([[5]])
print('The type of x is: ',type(x))
print('The size of x is: ',x.size())
print('The dimension of x is: ',x.ndim)
y = torch.det(x)
print('The determinant of x is y: ',y)
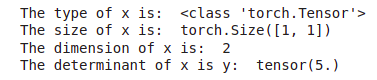
x = torch.Tensor([[5,1],
[1,5]])
print('The type of x is: ',type(x))
print('The size of x is: ',x.size())
print('The dimension of x is: ',x.ndim)
y = torch.det(x)
print('The determinant of x is y: ',y)
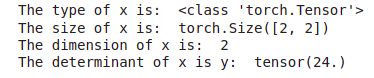
x = torch.Tensor([[[5,1],
[1,5]]])
print('The type of x is: ',type(x))
print('The size of x is: ',x.size())
print('The dimension of x is: ',x.ndim)
y = torch.det(x)
print('The determinant of x is y: ',y)
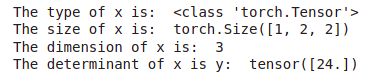
x = torch.Tensor([[[5,1],
[1,5]],
[[1,2],
[6,3]]
])
print('The type of x is: ',type(x))
print('The size of x is: ',x.size())
print('The dimension of x is: ',x.ndim)
y = torch.det(x)
print('The determinant of x is y: ',y)
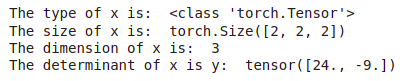
Indexing and slicing
x = torch.Tensor([5])
print('The type of x is: ',type(x))
print('The size of x is: ',x.size())
print('The dimension of x is: ',x.ndim)
x[0]
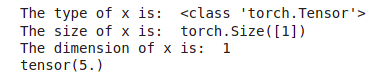
x = torch.Tensor([[5]])
print('The type of x is: ',type(x))
print('The size of x is: ',x.size())
print('The dimension of x is: ',x.ndim)
print(x[0])
print(x[0][0])
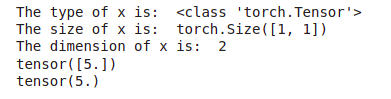
x = torch.Tensor([[5,1,2,3]])
print('The type of x is: ',type(x))
print('The size of x is: ',x.size())
print('The dimension of x is: ',x.ndim)
print(x[0])
print(x[0][0])
print(x[0][1])
print(x[0][:2])
print(x[0][-1])
print(x[0][2:4])
print(x[0][-3:])
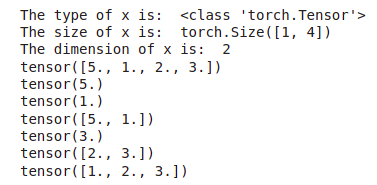
x = torch.Tensor([[5,1,2,3],
[1,5,6,9]])
print('The type of x is: ',type(x))
print('The size of x is: ',x.size())
print('The dimension of x is: ',x.ndim)
print('\nFirst row of tensor: \n',x[0],'\n')
print('First element of first row: \n',x[0][0])
print('\nSecond element of first row: \n',x[0][1])
print('\nFirst two elements of first row: \n',x[0][:2])
print('\nLast element of first row: \n',x[0][-1])
print('\n Second to forth (excluding) elements of first row:\n',x[0][2:4])
print('\n Last three elements of first row:\n',x[0][-3:])
print('\nSecond row of tensor: \n',x[1])
print('\nFirst element of second row: \n',x[1][0])
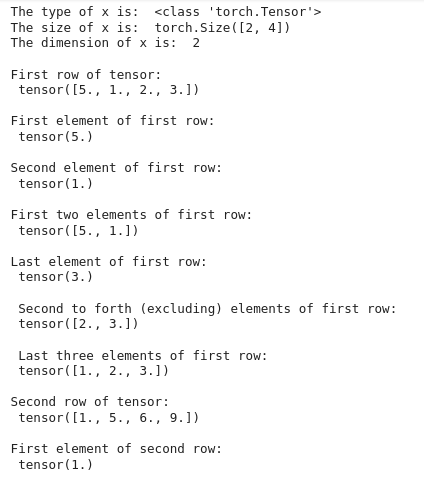
x = torch.Tensor([[5,1,2,3],
[1,5,6,9],
[1,2,3,4]])
print('The type of x is: ',type(x))
print('The size of x is: ',x.size())
print('The dimension of x is: ',x.ndim)
print('\nFirst column of tensor: \n',x[:,0],'\n')
print('\nLast column of tensor: \n',x[:,-1],'\n')
print('\nSecond and third column of tensor: \n',x[:,1:3],'\n')
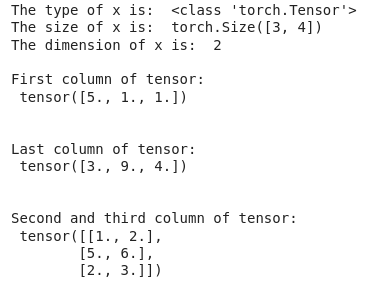
x = torch.Tensor([[[5,1,2,3],
[1,5,6,9],
[1,2,3,4]]])
print('The type of x is: ',type(x))
print('The size of x is: ',x.size())
print('The dimension of x is: ',x.ndim)
print('\nFirst column of tensor: \n',x[:,:,0],'\n')
print('\nLast column of tensor: \n',x[:,:,-1],'\n')
print('\nSecond and third column of tensor: \n',x[:,:,1:3],'\n')
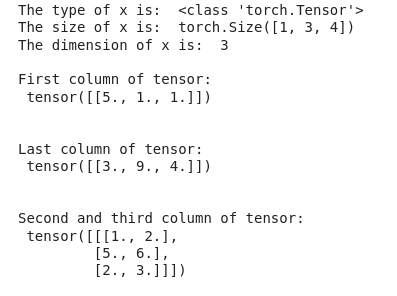
x = torch.Tensor([
[[5,1,2,3],
[1,5,6,9],
[1,2,3,4]],
[[1,5,6,1],
[1,8,9,0],
[1,2,3,6]],
[[1,1,1,0],
[2,2,2,0],
[0,0,0,0]]
])
print('The type of x is: ',type(x))
print('The size of x is: ',x.size())
print('The dimension of x is: ',x.ndim)
print('\n First matrix : \n',x[0])
print('\n Last matrix : \n',x[-1])
print('\n First row of all matrices : \n',x[:,0])
print('\n First and second row of all matrices : \n',x[:,0:2])
print('\n First and second column of all matrices : \n',x[:,:,0:2])
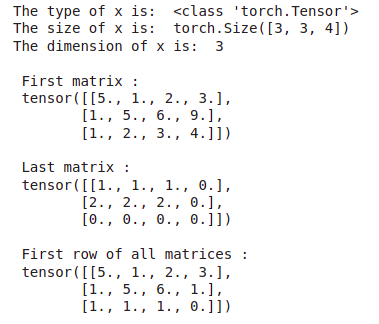
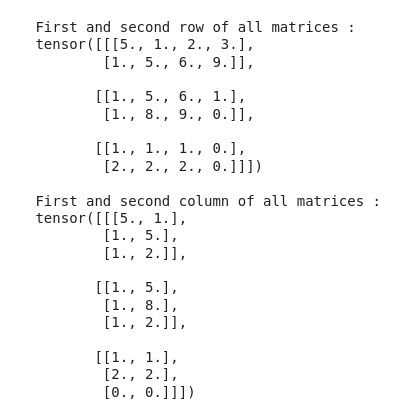
Broadcasting
x = torch.Tensor([5,5])
y = torch.Tensor([1])
print('The type of x is: ',type(x))
print('The size of x is: ',x.size())
print('The dimension of x is: ',x.ndim)
print('The type of y is: ',type(y))
print('The size of y is: ',y.size())
print('The dimension of y is: ',y.ndim)
print('Value of y will be added to both element of x\n')
print(x+y)
print((x+y).size())
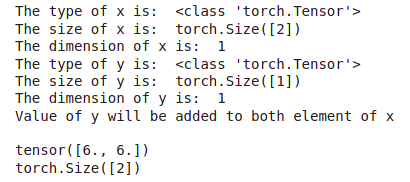
x = torch.Tensor([[5,5]])
y = torch.Tensor([1])
print('The type of x is: ',type(x))
print('The size of x is: ',x.size())
print('The dimension of x is: ',x.ndim)
print('The type of y is: ',type(y))
print('The size of y is: ',y.size())
print('The dimension of y is: ',y.ndim)
print('Value of y will be added to both element of x\n')
print(x+y)
print((x+y).size())
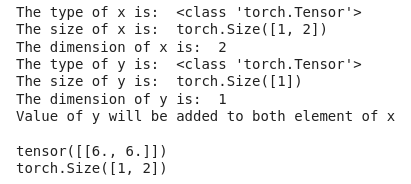
x = torch.Tensor([[5,5],
[0,0]])
y = torch.Tensor([1])
print('The type of x is: ',type(x))
print('The size of x is: ',x.size())
print('The dimension of x is: ',x.ndim)
print('\nThe type of y is: ',type(y))
print('The size of y is: ',y.size())
print('The dimension of y is: ',y.ndim)
print('Value of y will be added to all elements of x\n')
print(x+y)
print((x+y).size())
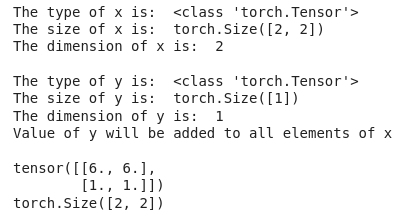
x = torch.Tensor([[5,5],
[0,0]])
y = torch.Tensor([1,2])
print('The type of x is: ',type(x))
print('The size of x is: ',x.size())
print('The dimension of x is: ',x.ndim)
print('\nThe type of y is: ',type(y))
print('The size of y is: ',y.size())
print('The dimension of y is: ',y.ndim)
print('Value of y will be added to all elements of x\n')
print(x+y)
print((x+y).size())
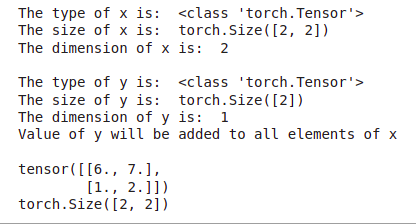
x = torch.Tensor([[[5,5],
[0,0]]])
y = torch.Tensor([1,2])
print('The type of x is: ',type(x))
print('The size of x is: ',x.size())
print('The dimension of x is: ',x.ndim)
print('\nThe type of y is: ',type(y))
print('The size of y is: ',y.size())
print('The dimension of y is: ',y.ndim)
print('Value of y will be added to all elements of x\n')
print(x+y)
print((x+y).size())
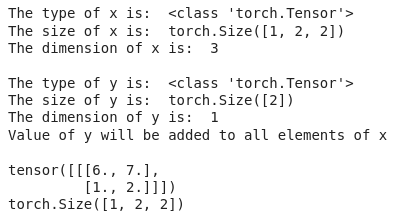
x = torch.Tensor([[[5,5],
[0,0]],
[[6,6],
[0,0]],
])
y = torch.Tensor([[1,1],
[1,1]])
print('The type of x is: ',type(x))
print('The size of x is: ',x.size())
print('The dimension of x is: ',x.ndim)
print('\nThe type of y is: ',type(y))
print('The size of y is: ',y.size())
print('The dimension of y is: ',y.ndim)
print('Value of y will be added to all matrices of x\n')
print(x+y)
print((x+y).size())
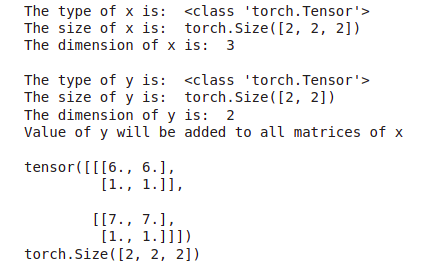
Reshape
v = torch.arange(9)
print(v)
v = v.view(3, 3)
print(v)
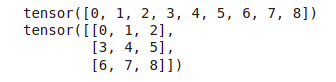
x = torch.rand(4,3)
print(x)
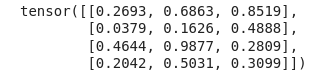
x.view(2,6)

Squeeze and Unsqueeze
x = torch.Tensor([[[5,5,6,6,7,8]]])
print('The type of x is: ',type(x))
print('The size of x is: ',x.size())
print('The dimension of x is: ',x.ndim)

y = torch.squeeze(x)
print('size of y is : ',y.size())
print('Dimension of y is: ',y.ndim)

x = torch.Tensor([[[5,5,6,6,7,8],
[1,4,7,8,5,6]]])
print('The type of x is: ',type(x))
print('The size of x is: ',x.size())
print('The dimension of x is: ',x.ndim)

y = torch.squeeze(x)
print(y)
print('\nsize of y is : ',y.size())
print('\nDimension of y is: ',y.ndim)
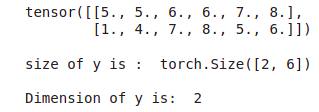
y = torch.squeeze(x,0)
print(y)
print('\nsize of y is : ',y.size())
print('\nDimension of y is: ',y.ndim)
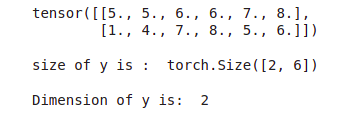
y = torch.squeeze(x,1)
print(y)
print('\nsize of y is : ',y.size())
print('\nDimension of y is: ',y.ndim)
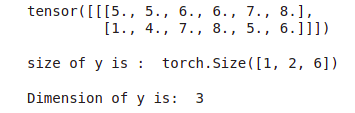
z = torch.unsqueeze(y,0)
print(z)
print('\nsize of z is : ',z.size())
print('\nDimension of z is: ',z.ndim)
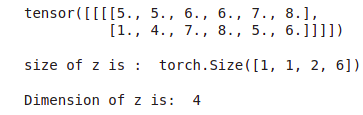
z = torch.unsqueeze(y,2)
print(z)
print('\nsize of z is : ',z.size())
print('\nDimension of z is: ',z.ndim)
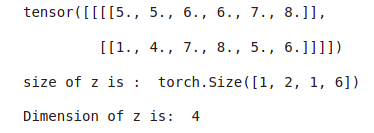
Concatenate and stack
x = torch.Tensor([5,5])
y = torch.Tensor([3,3])
print(torch.cat((x, y), 0))

x = torch.Tensor([[5,5],
[5,5]
])
y = torch.Tensor([[3,3],
[3,3]])
print(torch.cat((x, y), 0))
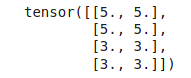
print(torch.cat((x, y),1))

print(torch.cat((x, y)))
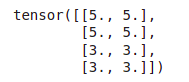
# Stack
print(torch.stack((x, y)))
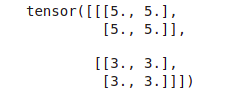
Reorganize the data element
v = torch.arange(9)
v = v.view(3, 3)
print(v)
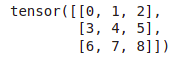
#torch.gather creates a new tensor from the input tensor by taking the values from each row along the input dimension dim.
# The values in torch.LongTensor, passed as index, specify which value to take from each 'row'.
# The dimension of the output tensor is same as the dimension of index tensor.
r = torch.gather(v, 1, torch.LongTensor([[0,1],[1,0],[2,1]]))
print(r)
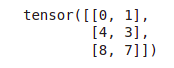
r = torch.chunk(v, 3)
print(r)

r = torch.split(v, 2)
print(r)

r = torch.split(v, 3)
print(r)
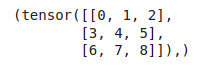
r = torch.split(v, 1)
print(r)

v = torch.rand(3,4)
print(v)
r = torch.split(v, 1)
print(r)

v = torch.rand(3,4)
print(v)
print('\n')
r = torch.split(v, 2)
print(r)

v = torch.rand(3,4)
print(v)
print('\n')
r = torch.split(v, 2,dim=0)
print(r)

v = torch.rand(3,4)
print(v)
print('\n')
r = torch.split(v, 2,dim=1)
print(r)
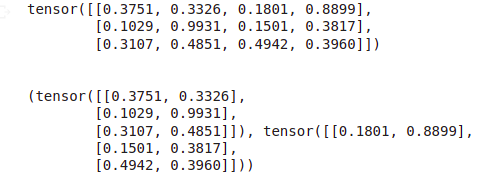
numpy and pytorch tensor
import numpy as np
x = np.array([2,3])
print(x)
print('\n')
t = torch.from_numpy(x)
print(t)
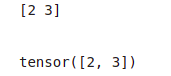
x = torch.Tensor([[5,5,6],
[8,9,6]])
print(x)
print(type(x))
print('\n')
print(x.numpy())
print(type(x.numpy()))
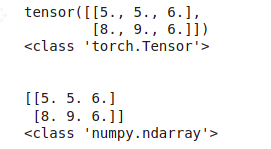
Operation on complex numbers
# create a complex tensor
a = torch.tensor([1+2j], dtype=torch.cfloat)
b = torch.tensor([2+3j], dtype=torch.cfloat)
print('The real part of a: \n',a.real)
print('\nThe imaginary part of a: \n',a.imag)
print('\n The absolute value of a: \n ',a.abs())
print('\n The angle of a: \n ',a.angle())
print('\n Result of addition of complex numbers:\n',a+b)
print('\n Result of subtraction of complex numbers:\n',a-b)
print('\n Result of multiplication of complex numbers:\n',a*b)
print('\n Result of division of complex numbers:\n',a/b)
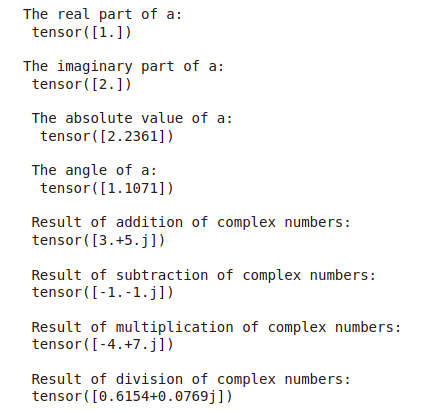
x = torch.Tensor([5,9])
print('Converting tensor of real numbers to tensor of complex number: \n',torch.view_as_complex(x))

a = torch.tensor([1+2j], dtype=torch.cfloat)
print('Converting tensor of complex number to tensor of real numbers : \n',torch.view_as_real(a))

pontu
0
Tags :