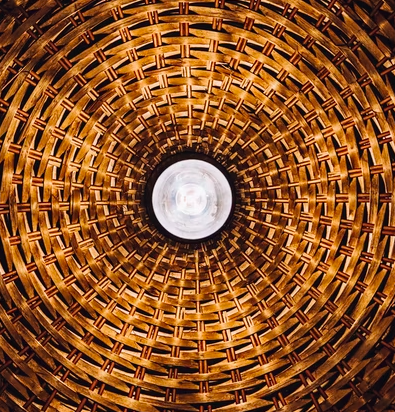
CNN and its friends in PyTorch
The blog about the CNN functions in PyTorch and other assisting functions generally used with Convolution Neural Networks.
Table of Contents
- One dimensional Convolution Neural Network
- Friends of Conv1d
- Normalization
- MaxPool1d
- Flatten
- A complete model with conv1d
- Two dimensional Convolution Neural Network
- Friends of Conv2d
- BatchNorm2d
- Maxpool
- A complete model of 2D CNN using PyTorch
- Combining CNN with BGRU
Basic import
import torch
import numpy as np
import torch
import torch.nn as nn
#Manual seed
torch.manual_seed(786)
One dimensional Convolution Neural Network
We generate a random data of 5×6
input = torch.rand(5,6)
Feed this data to Conv1d
Conv1d of PyTorch views the above input as a single data of array length 6 and 5 channels
We make a Conv1d network which takes a input of 5 channels and gives output of single channel
kernel size of the convolution filter is one
output = nn.Conv1d(5,1,1)(input)
print("The size of the input fed to conv1d: ",input.size())
print("\nThe size of the output produced by conv1d: ",output.size())
print("\nThe output produced by conv1d: ",output)
The size of the input fed to conv1d: torch.Size([5, 6])
The size of the output produced by conv1d: torch.Size([1, 6])
The output produced by conv1d: tensor([[-0.3618, -0.1509, -0.3991, -0.4515, -0.3065, -0.0403]], grad_fn=<SqueezeBackward1>)
Let us change the kernel size to 2
output = nn.Conv1d(5,1,2)(input)
print("The size of the input fed to conv1d: ",input.size())
print("\nThe size of the output produced by conv1d: ",output.size())
print("\nThe output produced by conv1d: ",output)
The size of the input fed to conv1d: torch.Size([5, 6])
The size of the output produced by conv1d: torch.Size([1, 5])
The output produced by conv1d: tensor([[ 0.0570, -0.0433, 0.0222, -0.0370, 0.1689]], grad_fn=<SqueezeBackward1>)
Let us have 2 output channels
output = nn.Conv1d(5,2,2)(input)
print("The size of the input fed to conv1d: ",input.size())
print("\nThe size of the output produced by conv1d: ",output.size())
print("\nThe output produced by conv1d: ",output)
The size of the input fed to conv1d: torch.Size([5, 6])
The size of the output produced by conv1d: torch.Size([2, 5])
The output produced by conv1d: tensor([[-0.6545, -0.4370, -0.4855, -0.6539, -0.9517], [ 0.2993, 0.3911, 0.0805, 0.1657, 0.3177]], grad_fn=<SqueezeBackward1>)
Let us feed 3d data
#We generate a random data of 5x6
input = torch.rand(1,5,6)
#Conv1d of PyTorch views the above input as a single data of array length 6 and 5 channels
output = nn.Conv1d(5,2,2)(input)
print("The size of the input fed to conv1d: ",input.size())
print("\nThe size of the output produced by conv1d: ",output.size())
print("\nThe output produced by conv1d: ",output)
The size of the input fed to conv1d: torch.Size([1, 5, 6])
The size of the output produced by conv1d: torch.Size([1, 2, 5])
The output produced by conv1d: tensor([[[-0.8972, -0.6160, -0.9812, -0.6427, -0.6077], [ 0.4216, 0.2899, 0.4674, 0.2571, 0.2153]]], grad_fn=<ConvolutionBackward0>)
input = torch.rand(2,5,6)
#Conv1d of PyTorch views the above input as a two different data of array length 6 and 5 channels
output = nn.Conv1d(5,2,2)(input)
print("The size of the input fed to conv1d: ",input.size())
print("\nThe size of the output produced by conv1d: ",output.size())
print("\nThe output produced by conv1d: ",output)
The size of the input fed to conv1d: torch.Size([2, 5, 6])
The size of the output produced by conv1d: torch.Size([2, 2, 5])
The output produced by conv1d: tensor([[[ 0.5094, 0.2010, 0.5080, 0.2805, 0.1527], [-0.1660, 0.1381, -0.0130, -0.1610, 0.2398]], [[ 0.2659, 0.1044, 0.2947, 0.1218, 0.0418], [ 0.0106, 0.1497, 0.0820, 0.0038, -0.0200]]], grad_fn=<ConvolutionBackward0>)
Adding stride
input = torch.rand(5,6)
output = nn.Conv1d(5,2,2,stride=1)(input)
print("The size of the input fed to conv1d: ",input.size())
print("\nThe size of the output produced by conv1d: ",output.size())
print("\nThe output produced by conv1d: ",output)
The size of the input fed to conv1d: torch.Size([5, 6])
The size of the output produced by conv1d: torch.Size([2, 5])
The output produced by conv1d: tensor([[-0.3402, -0.3649, -0.1783, -0.1039, -0.4152], [ 0.1544, 0.0834, -0.0204, 0.1661, -0.0524]], grad_fn=<SqueezeBackward1>)
input = torch.rand(5,6)
output = nn.Conv1d(5,2,2,stride=2)(input)
print("The size of the input fed to conv1d: ",input.size())
print("\nThe size of the output produced by conv1d: ",output.size())
print("\nThe output produced by conv1d: ",output)
The size of the input fed to conv1d: torch.Size([5, 6])
The size of the output produced by conv1d: torch.Size([2, 3])
The output produced by conv1d: tensor([[ 0.2920, 0.0630, -0.0170], [ 0.1152, 0.1165, -0.2402]], grad_fn=<SqueezeBackward1>)
Adding padding
output = nn.Conv1d(5,2,2,stride = 2,padding=2)(input)
print("The size of the output produced by conv1d: ",output.size())
print("The output produced by conv1d: ",output)
The size of the output produced by conv1d: torch.Size([2, 5])
The output produced by conv1d: tensor([[-0.2396, -0.4663, -0.3094, -0.0477, -0.2396], [ 0.1277, 0.0908, 0.1857, 0.1670, 0.1277]], grad_fn=<SqueezeBackward1>)
Friends of Conv1d
Normalization
#num_features – number of features or channels CC of the input
#We generate a random data of 5x6
input = torch.rand(5,6)
output = nn.BatchNorm1d(6)(input)
print("The size of the output produced by BatchNorm1d: ",output.size())
print("The output produced by BatchNorm1d: ",output)
The size of the output produced by conv1d: torch.Size([5, 6])
The output produced by conv1d: tensor([[-0.1792, -0.7942, 0.1733, 0.4468, -0.1337, -1.0347],
[ 1.6762, 1.5001, -1.2805, 0.5521, 0.4000, 1.0175],
[-0.3580, -1.3724, 1.7553, -1.1693, -0.3071, 1.3538],
[-1.4003, 0.2565, -0.4390, -1.1622, -1.5169, -0.3787],
[ 0.2613, 0.4099, -0.2092, 1.3327, 1.5576, -0.9578]],
grad_fn=<NativeBatchNormBackward0>)
#num_features – number of features or channels CC of the input
#We generate a random data of 5x6
input = torch.rand(1,5,6)
output = nn.BatchNorm1d(5)(input)
print("The size of the output produced by BatchNorm1d: ",output.size())
print("The output produced by BatchNorm1d: ",output)
The size of the output produced by BatchNorm1d: torch.Size([1, 5, 6])
The output produced by BatchNorm1d: tensor([[[ 1.7455e+00, -1.2547e-03, -2.5111e-02, 4.3375e-01, -6.0413e-01, -1.5487e+00], [ 7.3140e-01, -1.1725e+00, 1.0046e-01, -1.8527e-01, 1.6605e+00, -1.1346e+00], [-9.6463e-01, -9.8485e-01, 7.3110e-01, 1.1416e+00, 1.1008e+00, -1.0240e+00], [-4.7787e-01, 6.7520e-01, -1.2551e+00, 4.1689e-02, -7.6102e-01, 1.7771e+00], [-9.4955e-01, 9.4408e-01, 1.7738e+00, -5.6198e-01, -6.9480e-01, -5.1154e-01]]], grad_fn=<NativeBatchNormBackward0>)
Conv1d with BatchNorm1d
input = torch.rand(1,5,6)
output = nn.Conv1d(5,2,2,stride=2)(input)
output = nn.BatchNorm1d(2)(output)
print("The size of the output produced by BatchNorm1d: ",output.size())
print("The output produced by BatchNorm1d: ",output)
The size of the output produced by BatchNorm1d: torch.Size([1, 2, 3])
The output produced by BatchNorm1d: tensor([[[ 0.2846, -1.3417, 1.0570], [ 0.8692, -1.4002, 0.5310]]], grad_fn=<NativeBatchNormBackward0>)
MaxPool1d
torch.nn.MaxPool1d(kernel_size, stride=None, padding=0, dilation=1, return_indices=False, ceil_mode=False)
input = torch.rand(1,1,9)
print("The size of the input supplied to the maxpool1d: ",input.size())
print("The input itself: ",input)
#MaxPool1d with kernel size 3
output = nn.MaxPool1d(3)(input)
print("The size of the output produced by maxpool1d: ",output.size())
print("The output produced by maxpool1d: ",output)
The size of the input supplied to the maxpool1d torch.Size([1, 1, 9])
The input itself: tensor([[[0.2055, 0.0291, 0.6919, 0.8169, 0.7850, 0.0074, 0.7360, 0.9542, 0.2988]]])
The size of the output produced by maxpool1d: torch.Size([1, 1, 3])
The output produced by maxpool1d tensor([[[0.6919, 0.8169, 0.9542]]])
input = torch.rand(1,1,9)
#MaxPool1d with kernel size 2
output = nn.MaxPool1d(2)(input)
print("The size of the output produced by maxpool1d: ",output.size())
print("The output produced by maxpool1d: ",output)
The size of the output produced by maxpool1d: torch.Size([1, 1, 4])
The output produced by maxpool1d: tensor([[[0.9647, 0.7835, 0.2206, 0.6484]]])
input = torch.rand(1,1,9)
#MaxPool1d with kernel size 2 and stride of 1
output = nn.MaxPool1d(2,stride=1)(input)
print("The size of the output produced by maxpool1d: ",output.size())
print("The output produced by maxpool1d: ",output)
The size of the output produced by maxpool1d: torch.Size([1, 1, 8])
The output produced by maxpool1d: tensor([[[0.3641, 0.7910, 0.9314, 0.9314, 0.3826, 0.9551, 0.9551, 0.2818]]])
input = torch.rand(1,1,20)
#MaxPool1d with kernel size 4, stride of 1 with padding =2
output = nn.MaxPool1d(4,stride=1,padding=2)(input)
print("The size of the output produced by maxpool1d: ",output.size())
print("The output produced by maxpool1d: ",output)
The size of the output produced by maxpool1d: torch.Size([1, 1, 21])
The output produced by maxpool1d: tensor([[[0.6768, 0.7337, 0.7378, 0.7378, 0.7378, 0.7378, 0.4579, 0.4579, 0.4575, 0.8782, 0.9574, 0.9574, 0.9574, 0.9574, 0.7578, 0.7578, 0.8302, 0.8302, 0.8302, 0.8302, 0.7338]]])
Conv1d + MaxPool1d
input = torch.rand(1,5,20)
output = nn.Conv1d(5,1,2,stride=2)(input)
print("The size of the output produced by Conv1d: ",output.size())
print("\nThe output produced by Conv1d: ",output)
output = nn.MaxPool1d(4,stride=1,padding=2)(output)
print("\nThe size of the output produced by MaxPool1d: ",output.size())
print("\nThe output produced by MaxPool1d: ",output)
The size of the output produced by Conv1d: torch.Size([1, 1, 10])
The output produced by Conv1d: tensor([[[-0.0664, -0.2739, -0.1482, -0.3231, -0.2197, -0.1906, -0.3427, -0.0377, -0.2237, -0.0992]]], grad_fn=<ConvolutionBackward0>)
The size of the output produced by MaxPool1d: torch.Size([1, 1, 11])
The output produced by MaxPool1d: tensor([[[-0.0664, -0.0664, -0.0664, -0.1482, -0.1482, -0.1906, -0.0377, -0.0377, -0.0377, -0.0377, -0.0992]]], grad_fn=<SqueezeBackward1>)
input = torch.rand(1,5,60)
output = nn.Conv1d(5,2,2,stride=2)(input)
print("The size of the output produced by Conv1d: ",output.size())
output = nn.MaxPool1d(4,stride=1,padding=2)(output)
print("\nThe size of the output produced by MaxPool1d: ",output.size())
The size of the output produced by Conv1d: torch.Size([1, 2, 30])
The size of the output produced by MaxPool1d: torch.Size([1, 2, 31])
Flatten
input = torch.rand(1,1,9)
print("The size of the input supplied : ",input.size())
print("The input itself: ",input)
output = nn.Flatten()(input)
print("The size of the output produced by Flatten: ",output.size())
print("The output produced by Flatten: ",output)
The size of the input supplied : torch.Size([1, 1, 9])
The input itself: tensor([[[0.6603, 0.2366, 0.0950, 0.2664, 0.5605, 0.0224, 0.9319, 0.9204, 0.4543]]])
The size of the output produced by Flatten: torch.Size([1, 9])
The output produced by Flatten: tensor([[0.6603, 0.2366, 0.0950, 0.2664, 0.5605, 0.0224, 0.9319, 0.9204, 0.4543]])
input = torch.rand(1,2,9)
print("The size of the input supplied : ",input.size())
print("The input itself: ",input)
output = nn.Flatten()(input)
print("The size of the output produced by Flatten: ",output.size())
print("The output produced by Flatten: ",output)
The size of the input supplied : torch.Size([1, 2, 9])
The input itself: tensor([[[0.5552, 0.7913, 0.2304, 0.3114, 0.7368, 0.8721, 0.3572, 0.9759, 0.3256], [0.5029, 0.4612, 0.2495, 0.1843, 0.5261, 0.7532, 0.1901, 0.7778, 0.9928]]])
The size of the output produced by Flatten: torch.Size([1, 18])
The output produced by Flatten: tensor([[0.5552, 0.7913, 0.2304, 0.3114, 0.7368, 0.8721, 0.3572, 0.9759, 0.3256, 0.5029, 0.4612, 0.2495, 0.1843, 0.5261, 0.7532, 0.1901, 0.7778, 0.9928]])
input = torch.rand(2,2,2)
print("The size of the input supplied : ",input.size())
print("The input itself: ",input)
output = nn.Flatten()(input)
print("The size of the output produced by Flatten: ",output.size())
print("The output produced by Flatten: ",output)
The size of the input supplied : torch.Size([2, 2, 2])
The input itself: tensor([[[0.9673, 0.6878], [0.1287, 0.7666]], [[0.6812, 0.7579], [0.8650, 0.2871]]])
The size of the output produced by Flatten: torch.Size([2, 4])
The output produced by Flatten: tensor([[0.9673, 0.6878, 0.1287, 0.7666], [0.6812, 0.7579, 0.8650, 0.2871]])
Flattening the Conv1d
input = torch.rand(1,5,60)
output = nn.Conv1d(5,2,2,stride=2)(input)
print("The size of the output produced by Conv1d: ",output.size())
output = nn.Flatten()(output)
print("\nThe size of the output produced by Flatten: ",output.size())
The size of the output produced by Conv1d: torch.Size([1, 2, 30])
The size of the output produced by Flatten: torch.Size([1, 60])
A complete model with conv1d
model_conv1d_linear = torch.nn.Sequential(
torch.nn.Conv1d(6, 8, 3), #input layers = 6 output layers = 8 kernel of size = 3
torch.nn.BatchNorm1d(8),
torch.nn.MaxPool1d(2),
torch.nn.ReLU(),
torch.nn.Flatten(),
torch.nn.Linear(8,8),
torch.nn.ReLU(),
torch.nn.Linear(8,8),
torch.nn.ReLU(),
torch.nn.Linear(8, 1),
torch.nn.Sigmoid()
)
#Loss function
loss_func = nn.MSELoss()
#setting Stochastic gradient descent as an optimizer
from torch.optim import SGD
opt = SGD(model_conv1d_linear.parameters(),lr=0.001)
#Preparing the input and output
input = torch.randn(100,6,5) #100 data of array size 5 with 6 channels
print('The size of input: ',input.size())
t = torch.randn(100,1).uniform_(0,1)
output_data = torch.bernoulli(t)
print("The output data: \n",output_data.size())
x = input
y = output_data
Training the model
import time
loss_history = []
ticks = time.time()
epochs = 10000
for _ in range(epochs):
opt.zero_grad()
loss_value = loss_func(model_conv1d_linear(x),y)
loss_value.backward()
opt.step()
loss_value = loss_value.cpu().data.numpy()
loss_history.append(loss_value)
toc = time.time()
print("\n time taken: {}".format(toc-ticks))
import matplotlib.pyplot as plt
%matplotlib inline
plt.plot(loss_history)
plt.title('Loss variation over increasing epochs')
plt.xlabel('epochs')
plt.ylabel('loss values')
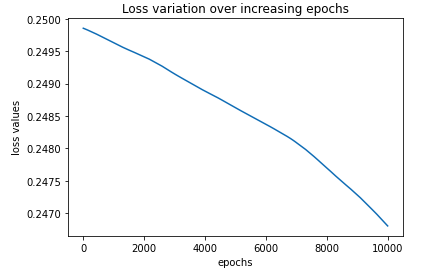
Two dimensional Convolution Neural Network
We generate a random data of 1×5×6
input = torch.rand(1,5,6)
Feed this data to Conv2d
Conv2d of PyTorch views the above input as a single data of 5×6
We make a Conv2d network which takes a input of 1 channel and gives output of single channel
kernel size of the convolution filter is one
output = nn.Conv2d(1,1,1)(input)
print("\nThe size of the output produced by conv2d: ",output.size())
print("\nThe output produced by conv2d: ",output)
The size of the output produced by conv2d: torch.Size([1, 5, 6])
The output produced by conv2d: tensor([[[0.7188, 0.6869, 0.7203, 0.7268, 0.6768, 0.7296], [0.6294, 0.9380, 0.7200, 0.7148, 0.7804, 0.7194], [0.8164, 0.8225, 0.7545, 0.9353, 0.6748, 0.7784], [0.7287, 0.8276, 0.8807, 0.9415, 0.7270, 0.9261], [0.7764, 0.8347, 0.6774, 0.7070, 0.8765, 0.7180]]], grad_fn=<SqueezeBackward1>)
Conv2d takes 3D or 4D data
Following code will generate error
input = torch.rand(5,6)
#no of in channels 1
#no of out channels 1
#kernel size 1
print("The size of the input supplied to the Conv2d: ",input.size())
output = nn.Conv2d(1,1,1)(input)
print("\nThe size of the output produced by conv2d: ",output.size())
print("\nThe output produced by conv2d: ",output)
Changing kernel size to 2
input = torch.rand(1,5,6)
#no of in channels 1
#no of out channels 1
#kernel size 2
#Here the Conv2d viwes the input as a single data of 5x6 with single layer
output = nn.Conv2d(1,1,2)(input)
print("\nThe size of the output produced by conv2d: ",output.size())
print("\nThe output produced by conv2d: ",output)
The size of the output produced by conv2d: torch.Size([1, 4, 5])
The output produced by conv2d: tensor([[[0.4550, 0.1860, 0.2722, 0.3107, 0.4229], [0.5645, 0.5351, 0.8032, 0.4268, 0.7535], [0.6573, 0.5700, 0.5821, 0.6744, 0.2662], [0.4018, 0.3585, 0.3007, 0.4316, 0.5243]]], grad_fn=<SqueezeBackward1>)
Conv2d with two output channels
#random data of 1x5x6
input = torch.rand(1,5,6)
#no of in channels 1
#no of out channels 2
#kernel size 2
#Here the Conv2d viwes the input as a single data of 5x6 with single layer
output = nn.Conv2d(1,2,2)(input)
print("\nThe size of the output produced by conv2d: ",output.size())
print("\nThe output produced by conv2d: ",output)
The size of the output produced by conv2d: torch.Size([2, 4, 5])
The output produced by conv2d: tensor([[[ 0.1826, 0.2502, 0.1240, 0.0748, 0.0219], [ 0.0161, 0.0197, 0.1369, 0.5326, 0.1054], [ 0.1373, 0.2973, -0.0486, 0.1999, 0.0332], [-0.0191, -0.1692, 0.4239, 0.1180, 0.1320]], [[-0.7733, -0.7674, -0.7013, -0.7786, -0.6286], [-0.7498, -0.6347, -0.7375, -0.9912, -0.7727], [-0.6833, -0.7271, -0.7864, -0.7889, -0.6613], [-0.6974, -0.6065, -0.8430, -0.7241, -0.6897]]], grad_fn=<SqueezeBackward1>)
Rectangular kernel
input = torch.rand(1,5,6)
#kernel size (2,3)
output = nn.Conv2d(1,2,kernel_size = (2,3))(input)
print("\nThe size of the output produced by conv2d: ",output.size())
print("\nThe output produced by conv2d: ",output)
The size of the output produced by conv2d: torch.Size([2, 4, 4])
The output produced by conv2d: tensor([[[-0.2476, -0.4501, -0.4143, -0.4086], [-0.3632, -0.3321, -0.3738, -0.2921], [-0.1048, -0.1158, -0.1017, -0.2735], [-0.4860, -0.3348, -0.3935, -0.3509]], [[-0.0252, -0.2791, -0.1702, -0.0584], [-0.2167, -0.0783, -0.2385, -0.2000], [-0.0534, -0.2682, -0.1249, -0.1798], [-0.2714, -0.0045, -0.0737, -0.1698]]], grad_fn=<SqueezeBackward1>)
Padding
input = torch.rand(1,5,6)
#padding = 2
output = nn.Conv2d(1,1,2,padding=2)(input)
print("\nThe size of the output produced by conv2d: ",output.size())
print("\nThe output produced by conv2d: ",output)
The size of the output produced by conv2d: torch.Size([1, 8, 9])
The output produced by conv2d: tensor([[[ 0.3255, 0.3255, 0.3255, 0.3255, 0.3255, 0.3255, 0.3255, 0.3255, 0.3255], [ 0.3255, 0.3989, 0.5906, 0.6741, 0.6129, 0.6330, 0.7266, 0.5700, 0.3255], [ 0.3255, 0.2662, 0.2260, 0.2495, 0.3007, 0.1131, -0.1562, 0.1066, 0.3255], [ 0.3255, 0.2556, 0.0937, -0.1613, -0.1567, 0.0319, 0.2785, 0.4675, 0.3255], [ 0.3255, 0.1153, 0.0968, 0.3590, 0.4502, 0.4476, 0.4834, 0.3763, 0.3255], [ 0.3255, 0.2854, 0.3502, 0.0904, 0.0900, 0.1767, -0.1759, 0.0826, 0.3255], [ 0.3255, 0.0018, -0.2464, -0.0229, -0.0415, -0.0844, 0.0092, 0.1506, 0.3255], [ 0.3255, 0.3255, 0.3255, 0.3255, 0.3255, 0.3255, 0.3255, 0.3255, 0.3255]]], grad_fn=<SqueezeBackward1>)
Padding =(2,3)
input = torch.rand(1,5,6)
#padding = (2,3)
output = nn.Conv2d(1,1,2,padding=(2,3))(input)
print("\nThe size of the output produced by conv2d: ",output.size())
The size of the output produced by conv2d: torch.Size([1, 8, 11])
Feeding the data with three input channels
input = torch.rand(3,5,6)
#no of in channels 3
#no of out channels 2
#kernel size 2
print("The size of the input supplied to the Conv2d: ",input.size())
#Here the Conv2d viwes the input as a single data of 5x6 with Three channels
output = nn.Conv2d(3,2,2)(input)
print("\nThe size of the output produced by conv2d: ",output.size())
The size of the input supplied to the Conv2d: torch.Size([3, 5, 6])
The size of the output produced by conv2d: torch.Size([2, 4, 5])
Rectangular Kernel and unequal stride
#Rectangular kernel
#unequal stride
input = torch.rand(1,5,6)
print("The size of the input supplied to the conv2d: ",input.size())
output = nn.Conv2d(1,1,(2,3),(2,1),2)(input)
print("The size of the output produced by conv2d: ",output.size())
The size of the input supplied to the conv2d: torch.Size([1, 5, 6])
The size of the output produced by conv2d: torch.Size([1, 4, 8])
Rectangular Kernel, unequal stride and unequal padding
#Rectangular kernel
#unequal stride
#unequal padding
input = torch.rand(1,5,6)
print("The size of the input supplied to the conv2d: ",input.size())
output = nn.Conv2d(1,1,(2,3),(2,1),(2,1))(input)
print("The size of the output produced by conv2d: ",output.size())
The size of the input supplied to the conv2d: torch.Size([1, 5, 6])
The size of the output produced by conv2d: torch.Size([1, 4, 6])
Feeding 4d data
#Rectangular kernel
#unequal stride
#unequal padding
#Feeding 4d data
#Conv2d sees this data as a batch of 4
#Where each data is 5x6 with single channel
input = torch.rand(4,1,5,6)
print("The size of the input supplied to the conv2d: ",input.size())
output = nn.Conv2d(1,1,(2,3),(2,1),(2,1))(input)
print("The size of the output produced by conv2d: ",output.size())
The size of the input supplied to the conv2d: torch.Size([4, 1, 5, 6])
The size of the output produced by conv2d: torch.Size([4, 1, 4, 6])
Friends of Conv2d
BatchNorm2d
input = torch.rand(1,1,10,10)
#no of chanels of input =1
#this is supplied as parameter of BatchNorm2d
output = nn.BatchNorm2d(1)(input)
print("The size of the output produced by BatchNorm2d: ",output.size())
The size of the output produced by BatchNorm2d: torch.Size([1, 1, 10, 10])
input = torch.rand(1,2,10,10)
#no of chanels of input =2
#this is supplied as parameter of BatchNorm2d
output = nn.BatchNorm2d(2)(input)
print("The size of the output produced by BatchNorm2d: ",output.size())
The size of the output produced by BatchNorm2d: torch.Size([1, 2, 10, 10])
input = torch.rand(5,2,3,3)
#no of chanels of input =2
#this is supplied as parameter of BatchNorm2d
output = nn.BatchNorm2d(2)(input)
print("The size of the output produced by BatchNorm2d: ",output.size())
The size of the output produced by BatchNorm2d: torch.Size([5, 2, 3, 3])
Conv2d + BatchNorm2d
input = torch.rand(1,1,10,10)
output = nn.Conv2d(1,2,2)(input)
print("The size of the output produced by conv2d: ",output.size())
output = nn.BatchNorm2d(2)(output)
print("The size of the output produced by BatchNorm2d: ",output.size())
The size of the output produced by conv2d: torch.Size([1, 2, 9, 9])
The size of the output produced by BatchNorm2d: torch.Size([1, 2, 9, 9])
Maxpool
using kernel size of 2 which means it finds max value of 2×2
input = torch.rand(1,1,4,4)
print("the input: ",input)
#using kernel size of 2 which means it finds max value of 2x2
output = nn.MaxPool2d(2)(input)
print("\n the output: ",output)
the input: tensor([[[[0.9620, 0.2912, 0.9606, 0.3570], [0.8146, 0.3626, 0.6013, 0.5377], [0.6888, 0.5530, 0.8818, 0.1461], [0.1774, 0.2611, 0.3099, 0.5475]]]])
the output: tensor([[[[0.9620, 0.9606], [0.6888, 0.8818]]]]
input = torch.rand(1,1,7,7)
print("the input: ",input)
#using kernel size of 4 which means it finds max value of 4x4 window
output = nn.MaxPool2d(4)(input)
print("\n the output: ",output)
the input: tensor([[[[0.0400, 0.0778, 0.2094, 0.7854, 0.7714, 0.2330, 0.4067], [0.1511, 0.2139, 0.6737, 0.5671, 0.3439, 0.1558, 0.0686], [0.6684, 0.3254, 0.0722, 0.3005, 0.9211, 0.3858, 0.2834], [0.4717, 0.3984, 0.5096, 0.0373, 0.8166, 0.2719, 0.6958], [0.1651, 0.0932, 0.5495, 0.1845, 0.9775, 0.8319, 0.6891], [0.5360, 0.8048, 0.2986, 0.2762, 0.2960, 0.7120, 0.4551], [0.2162, 0.2306, 0.0093, 0.0686, 0.2996, 0.9747, 0.5090]]]])
the output: tensor([[[[0.7854]]]])
Kernel size =4 and padding = 2
input = torch.rand(1,1,7,7)
print("the input: ",input)
output = nn.MaxPool2d(4,padding=2)(input)
print("\n the output: ",output)
the input: tensor([[[[0.5974, 0.3705, 0.3844, 0.0491, 0.8386, 0.5553, 0.5883], [0.5310, 0.6815, 0.8516, 0.3602, 0.9677, 0.7994, 0.5934], [0.9562, 0.9477, 0.3423, 0.4497, 0.7911, 0.2446, 0.8893], [0.6198, 0.5478, 0.9720, 0.6601, 0.5098, 0.9914, 0.4262], [0.6138, 0.5181, 0.6620, 0.1366, 0.4421, 0.2970, 0.7821], [0.3284, 0.6922, 0.8223, 0.4193, 0.6871, 0.3830, 0.6815], [0.5241, 0.3626, 0.2737, 0.2560, 0.3880, 0.4218, 0.6155]]]])
the output: tensor([[[[0.6815, 0.9677],0.9562, 0.9914]]]])
Conv2d + MaxPool2d
input = torch.rand(1,1,10,10)
output = nn.Conv2d(1,1,2)(input)
print("The size of Conv2d output is: ",output.size())
output = nn.MaxPool2d(4,padding=2)(output)
print("The size of MaxPool2d output is: ",output.size())
The size of Conv2d output is: torch.Size([1, 1, 9, 9])
The size of MaxPool2d output is: torch.Size([1, 1, 3, 3])
A complete model of 2D CNN using PyTorch
model_conv2d_linear = torch.nn.Sequential(
torch.nn.Conv2d(3, 3, 3, stride=2),
torch.nn.BatchNorm2d(3),
torch.nn.MaxPool2d(2, stride=1),
torch.nn.ReLU(),
torch.nn.Flatten(),
torch.nn.Linear(36,20),
torch.nn.ReLU(),
torch.nn.Linear(20,8),
torch.nn.ReLU(),
torch.nn.Linear(8, 3),
torch.nn.Softmax()
)
#Loss function
loss_func = nn.MSELoss()
#setting Stochastic gradient descent as an optimizer
from torch.optim import SGD
opt = SGD(model_conv2d_linear.parameters(),lr=0.01)
#Preparing the input and output
input = torch.randn(1000,3,10,12) #100 data of size 10x10 with single channel
print('The size of input: ',input.size())
t = torch.randn(1000,1).uniform_(0,1)
output_data = torch.bernoulli(t)
print("The output data: \n",output_data.size())
x = input
y = output_data
loss_history = []
epochs = 1000
ticks = time.time()
for _ in range(epochs):
opt.zero_grad()
loss_value = loss_func(model_conv2d_linear(x),y)
loss_value.backward()
opt.step()
loss_value = loss_value.cpu().data.numpy()
loss_history.append(loss_value)
toc = time.time()
print("\n time taken: {}".format(toc-ticks))
import matplotlib.pyplot as plt
%matplotlib inline
plt.plot(loss_history)
plt.title('Loss variation over increasing epochs')
plt.xlabel('epochs')
plt.ylabel('loss values')
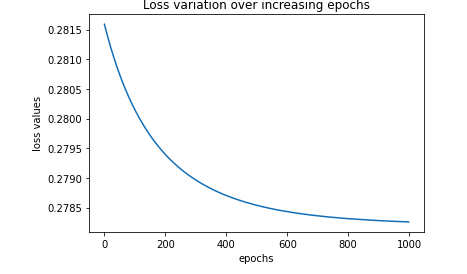
Combining CNN with BGRU
#helper function to extract the ouput of BGRU
class extract_tensor_2d_input(torch.nn.Module):
def forward(self,x):
tensor, _ = x
return tensor
model_conv2d_BGRU = torch.nn.Sequential(
torch.nn.Conv2d(1, 1, 2),
torch.nn.BatchNorm2d(1),
torch.nn.MaxPool2d(2),
torch.nn.ReLU(),
torch.nn.Flatten(),
torch.nn.GRU(20,12,bidirectional=True),
extract_tensor_2d_input(),
torch.nn.ReLU(),
torch.nn.Linear(24, 40),
torch.nn.ReLU(),
torch.nn.Linear(40,10),
torch.nn.ReLU(),
torch.nn.Linear(10, 1),
torch.nn.Sigmoid()
)
#Preparing the input and output
input = torch.randn(1000,1,10,12) #1000 data of size 10x12 with single channel
print('The size of input: ',input.size())
t = torch.randn(1000,1).uniform_(0,1)
output_data = torch.bernoulli(t)
print("The output data: \n",output_data.size())
x = input
y = output_data
#Loss function
loss_func = nn.MSELoss()
#setting Stochastic gradient descent as an optimizer
from torch.optim import SGD
opt = SGD(model_conv2d_BGRU.parameters(),lr=0.1)
loss_history = []
epochs = 100
ticks = time.time()
for _ in range(epochs):
opt.zero_grad()
loss_value = loss_func(model_conv2d_BGRU(x),y)
loss_value.backward()
opt.step()
loss_value = loss_value.cpu().data.numpy()
loss_history.append(loss_value)
toc = time.time()
print("\n time taken: {}".format(toc-ticks))
import matplotlib.pyplot as plt
%matplotlib inline
plt.plot(loss_history)
#plt.title('Loss variation over increasing epochs')
plt.xlabel('epochs')
plt.ylabel('loss values')
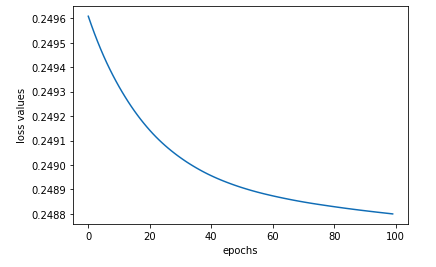