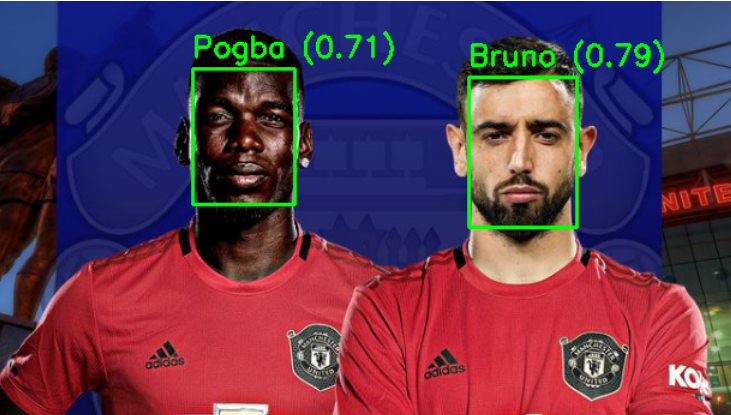
Face Detection and Recognition with Python, OpenCV, and Deep Learning: A Step-by-Step Guide
Face recognition technology has become an essential part of modern applications, from smartphone security to surveillance systems. In this step-by-step guide, we’ll walk you through building a powerful face detection and recognition system using Python, OpenCV, and deep learning. You’ll not only learn how to train your own model but also how to deploy it effectively on static images, pre-recorded videos, and even real-time webcam feeds. Whether you’re a beginner or an experienced developer looking to sharpen your skills, this tutorial will equip you with everything you need to implement face recognition in your own projects.
Table of contents
- Training
- Face Detection and Recognition on Image
- Face Detection and Recognition on Video
- Face Detection and Recognition on Live Stream
Training
The face recognition model training pipeline begins with data preparation, where images are loaded from a structured directory (DATA_DIR
), with each person’s images stored in separate folders. This organization allows the model to associate each image with a corresponding label based on the folder name.
In the face detection and preprocessing stage, each image is read using OpenCV and converted from BGR to RGB format to suit the requirements of the MTCNN face detector. MTCNN accurately locates and crops the face region from the image. If no face is detected, the image is skipped to maintain data integrity.
Next, in the feature extraction phase, the detected face is resized to 160×160 pixels and passed through the pre-trained InceptionResnetV1 model. This deep neural network, trained on the VGGFace2 dataset, transforms the face into a 512-dimensional embedding that captures unique facial features.
Once embeddings are collected, the model training step begins. Labels (person names) are encoded into numerical format using LabelEncoder
. These embeddings and encoded labels are used to train a Support Vector Machine (SVM) classifier with a linear kernel, which learns to distinguish between different individuals based on the face features.
Finally, in the model saving step, the trained SVM classifier and the label encoder are serialized using joblib
and saved to disk. This allows for the model to be loaded and used later for real-time face recognition tasks on new images or video streams.
Block Diagram
+----------------------------------------------------------+
| Load Image Dataset |
| (Images stored in labeled folders, one per person) |
+----------------------------------------------------------+
│
▼
+----------------------------------------------------------+
| Face Detection using MTCNN |
| - Detects face(s) in the image |
| - Applies a margin around the detected face |
| - Extracts the face region |
+----------------------------------------------------------+
│
▼
+----------------------------------------------------------+
| Face Preprocessing |
| - Converts to RGB format (for consistency) |
| - Resizes face to 160x160 pixels (required by model) |
+----------------------------------------------------------+
│
▼
+----------------------------------------------------------+
| Feature Extraction using InceptionResnetV1 |
| - Converts preprocessed face into a high-dimensional |
| vector (embedding) |
| - Embedding captures unique facial features |
| - Output: A 512-dimensional feature vector |
+----------------------------------------------------------+
│
▼
+----------------------------------------------------------+
| Label Encoding |
| - Converts person names into numerical labels |
| - Required for training SVM |
+----------------------------------------------------------+
│
▼
+----------------------------------------------------------+
| Train Classifier using Support Vector Machine |
| - Uses linear kernel to classify embeddings |
| - Learns decision boundaries for different faces |
| - Outputs probability scores for predictions |
+----------------------------------------------------------+
│
▼
+----------------------------------------------------------+
| Save Model (SVM Classifier + Label Encoder) |
| - Stores trained SVM for later face recognition |
| - Saves label encoder for decoding predictions |
+----------------------------------------------------------+
Code
#Import
import os
import torch
import cv2
from facenet_pytorch import MTCNN, InceptionResnetV1
from sklearn.preprocessing import LabelEncoder
from sklearn.svm import SVC
import joblib
# Paths
DATA_DIR = "face_dataset"
MODEL_PATH = "Models/face_recognition2.pkl"
# Load face detection and embedding models
device = torch.device("cuda" if torch.cuda.is_available() else "cpu")
mtcnn = MTCNN(image_size=160, margin=20, device=device)
resnet = InceptionResnetV1(pretrained="vggface2").eval().to(device)
# Lists to store face embeddings and labels
face_embeddings = []
labels = []
for person in os.listdir(DATA_DIR):
person_path = os.path.join(DATA_DIR, person)
for img_name in os.listdir(person_path):
img_path = os.path.join(person_path, img_name)
img = cv2.imread(img_path)
img_rgb = cv2.cvtColor(img, cv2.COLOR_BGR2RGB)
# Detect face
face = mtcnn(img_rgb)
if face is None:
continue # Skip if no face is detected
# Convert face to embedding
face = face.unsqueeze(0).to(device)
embedding = resnet(face).detach().cpu().numpy().flatten()
face_embeddings.append(embedding)
labels.append(person)
# Encode labels
encoder = LabelEncoder()
labels_encoded = encoder.fit_transform(labels)
# Train SVM classifier
clf = SVC(kernel="linear", probability=True)
clf.fit(face_embeddings, labels_encoded)
# Save model and label encoder
joblib.dump({"classifier": clf, "encoder": encoder}, MODEL_PATH)
print(f"Model saved as {MODEL_PATH}")
Face Detection and Recognition on Image
The recognize_faces
function is designed to perform face recognition on a given input image using a pre-trained face recognition pipeline. It starts by loading two core deep learning models: MTCNN for face detection and InceptionResnetV1 for extracting high-dimensional face embeddings. It also loads a previously trained Support Vector Machine (SVM) classifier and a LabelEncoder from disk using joblib
. After reading and converting the input image to RGB format, it uses MTCNN to detect faces within the image. For each detected face, the function crops and resizes it to 160×160 pixels to match the input size expected by the FaceNet model (InceptionResnetV1). The resized face is converted into a tensor, normalized, and passed through the model to obtain a 512-dimensional embedding. This embedding is then classified using the SVM to predict the person’s identity. If the prediction confidence (probability) is above 0.6, the corresponding label is shown; otherwise, the person is labeled “Unknown.” Finally, the function draws bounding boxes and labels on the image and displays the annotated result.
Block Diagram
┌────────────────────────────┐
│ Load Pretrained Models │
│ - MTCNN for detection │
│ - InceptionResnetV1 │
└────────────┬───────────────┘
│
▼
┌────────────────────────────┐
│ Load Trained Classifier │
│ - SVM Classifier │
│ - LabelEncoder │
└────────────┬───────────────┘
│
▼
┌────────────────────────────┐
│ Read Input Image │
│ Convert BGR → RGB │
└────────────┬───────────────┘
│
▼
┌────────────────────────────┐
│ Detect Faces using MTCNN │
│ (Returns bounding boxes) │
└────────────┬───────────────┘
│
▼
┌──────────────────────────────────────────────┐
│ For Each Detected Face: │
│ - Crop and resize to 160x160 │
│ - Convert to tensor and normalize │
│ - Get embedding via InceptionResnetV1 │
│ - Predict identity via SVM │
│ - If prob > 0.6, assign label │
│ - Draw bounding box + label on image │
└──────────────────────────────────────────────┘
│
▼
┌────────────────────────────┐
│ Display Final Annotated │
│ Image using PIL │
└────────────────────────────┘
Code
import torch
import numpy as np
import cv2
from facenet_pytorch import MTCNN, InceptionResnetV1
import joblib
from IPython.display import display
MODEL_PATH = "Models/face_recognition2.pkl"
IMAGE_PATH = "test_files/2.png"
def recognize_faces(input_img=IMAGE_PATH,MODEL_PATH=MODEL_PATH):
# Load face detection and embedding models
device = torch.device("cuda" if torch.cuda.is_available() else "cpu")
mtcnn = MTCNN(image_size=160, margin=20, device=device)
resnet = InceptionResnetV1(pretrained="vggface2").eval().to(device)
# Load trained classifier
data = joblib.load(MODEL_PATH)
clf = data["classifier"]
encoder = data["encoder"]
# Load test image
try:
img = cv2.imread(input_img)
img_rgb = cv2.cvtColor(img, cv2.COLOR_BGR2RGB)
except Exception as e:
print(f"Error: {e}")
# Detect faces
boxes, _ = mtcnn.detect(img_rgb)
if boxes is None:
print(" No face detected!")
else:
for i, box in enumerate(boxes):
x1, y1, x2, y2 = map(int, box)
# Crop and preprocess face
face = img_rgb[y1:y2, x1:x2] # Crop face
face = cv2.resize(face, (160, 160)) # Resize for FaceNet
face = torch.tensor(face).permute(2, 0, 1).float().unsqueeze(0) / 255.0
face = face.to(device)
# Extract face embedding
embedding = resnet(face).detach().cpu().numpy().flatten()
# Predict identity
probs = clf.predict_proba([embedding])[0]
max_prob = max(probs)
predicted_label = encoder.inverse_transform([np.argmax(probs)])[0]
# Draw bounding box and label
label_text = predicted_label if max_prob > 0.6 else "Unknown"
cv2.rectangle(img, (x1, y1), (x2, y2), (0, 255, 0), 2)
cv2.putText(img, f"{label_text} ({max_prob:.2f})", (x1, y1 - 10),
cv2.FONT_HERSHEY_SIMPLEX, 0.8, (0, 255, 0), 2)
image = cv2.cvtColor(img, cv2.COLOR_BGR2RGB)
display(PIL.Image.fromarray(image))
return None
recognize_faces(input_img=IMAGE_PATH)
Output
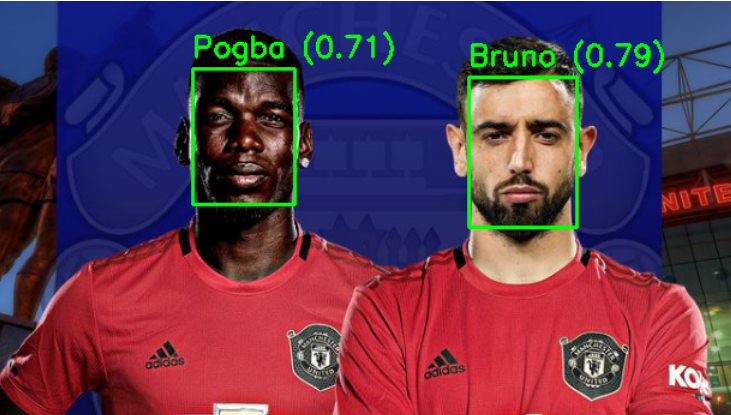
Face Detection and Recognition on Video
The process_video
function performs real-time face recognition on a video file using deep learning models and a pre-trained classifier. It begins by loading the MTCNN model for detecting faces in each frame and InceptionResnetV1 for generating 512-dimensional face embeddings. A previously trained SVM classifier and LabelEncoder are loaded using joblib
. The function reads the input video using OpenCV, retrieves frame properties like FPS, resolution, and total number of frames, and prepares to write the output video. For every frame, it detects faces using MTCNN, extracts and preprocesses each face, and then feeds it to the FaceNet model to get embeddings. These embeddings are passed to the SVM to predict identity. If the confidence is above 60%, the person’s name is displayed; otherwise, it’s labeled as “Unknown”. Bounding boxes and labels are drawn on the video, and recognition logs (timestamped) are saved to a text file. Finally, the processed video with annotations and logs is saved.
Block Diagram
┌────────────────────────────────────────────┐
│ Load Pretrained Models │
│ - MTCNN for face detection │
│ - InceptionResnetV1 for embeddings │
└──────────────────────┬─────────────────────┘
│
▼
┌────────────────────────────────────────────┐
│ Load Trained Classifier │
│ - SVM Classifier (joblib) │
│ - LabelEncoder │
└──────────────────────┬─────────────────────┘
│
▼
┌────────────────────────────────────────────┐
│ Read Input Video using OpenCV │
│ - Get FPS, resolution, total frames │
└──────────────────────┬─────────────────────┘
│
▼
┌────────────────────────────────────────────┐
│ For Each Frame in Video: │
│ - Convert BGR to RGB │
│ - Detect Faces using MTCNN │
└──────────────────────┬─────────────────────┘
│
▼
┌────────────────────────────────────────────────────────┐
│ For Each Detected Face: │
│ - Crop and resize to 160x160 │
│ - Normalize and convert to tensor │
│ - Generate embedding with InceptionResnetV1 │
│ - Predict identity using SVM │
│ - If prob > 0.6, assign label │
│ - Draw bounding box and label on frame │
│ - Log result with timestamp │
└──────────────────────┬─────────────────────────────────┘
│
▼
┌────────────────────────────────────────────┐
│ Write Annotated Frame to Output Video │
└──────────────────────┬─────────────────────┘
│
▼
┌────────────────────────────────────────────┐
│ After All Frames: │
│ - Save Final Video │
│ - Save Recognition Log │
└────────────────────────────────────────────┘
Code
import torch
import numpy as np
import cv2
from facenet_pytorch import MTCNN, InceptionResnetV1
import joblib
from datetime import timedelta
def process_video(video_path, output_video_path="Output/output.mp4", log_path="recognition_log.txt",MODEL_PATH=None):
device = torch.device("cuda" if torch.cuda.is_available() else "cpu")
mtcnn = MTCNN(image_size=160, margin=20, device=device)
resnet = InceptionResnetV1(pretrained="vggface2").eval().to(device)
data = joblib.load(MODEL_PATH)
clf = data["classifier"]
encoder = data["encoder"]
cap = cv2.VideoCapture(video_path)
# Read and verify FPS
fps = cap.get(cv2.CAP_PROP_FPS)
if fps == 0 or np.isnan(fps):
fps = 25.0 # Safe default
print("⚠️ FPS was 0 or invalid. Defaulting to 25 FPS.")
else:
print(f"🎞️ Detected FPS: {fps}")
width = int(cap.get(cv2.CAP_PROP_FRAME_WIDTH))
height = int(cap.get(cv2.CAP_PROP_FRAME_HEIGHT))
total_frames = int(cap.get(cv2.CAP_PROP_FRAME_COUNT))
duration_sec = total_frames / fps
print(f"🎥 Input video has {total_frames} frames, duration ~ {duration_sec:.2f} seconds.")
fourcc = cv2.VideoWriter_fourcc(*'mp4v')
out = cv2.VideoWriter(output_video_path, fourcc, fps, (width, height))
log = open(log_path, "w")
frame_idx = 0
while True:
ret, frame = cap.read()
if not ret:
break
img_rgb = cv2.cvtColor(frame, cv2.COLOR_BGR2RGB)
boxes, _ = mtcnn.detect(img_rgb)
if boxes is not None:
for box in boxes:
x1, y1, x2, y2 = map(int, box)
x1, y1 = max(x1, 0), max(y1, 0)
x2, y2 = min(x2, frame.shape[1]), min(y2, frame.shape[0])
face = img_rgb[y1:y2, x1:x2]
if face.size == 0:
continue
face_resized = cv2.resize(face, (160, 160))
face_tensor = torch.tensor(face_resized).permute(2, 0, 1).float().unsqueeze(0) / 255.0
face_tensor = face_tensor.to(device)
with torch.no_grad():
embedding = resnet(face_tensor).cpu().numpy().flatten()
probs = clf.predict_proba([embedding])[0]
max_prob = max(probs)
label = encoder.inverse_transform([np.argmax(probs)])[0] if max_prob > 0.6 else "Unknown"
cv2.rectangle(frame, (x1, y1), (x2, y2), (0, 255, 0), 2)
cv2.putText(frame, f"{label} ({max_prob:.2f})", (x1, y1 - 10),
cv2.FONT_HERSHEY_SIMPLEX, 0.7, (0, 255, 0), 2)
timestamp = str(timedelta(seconds=frame_idx / fps))
log.write(f"[{timestamp}] {label} ({max_prob:.2f})\n")
out.write(frame)
frame_idx += 1
cap.release()
out.release()
log.close()
output_duration = frame_idx / fps
print(f"Finished. Total frames written: {frame_idx} (~{output_duration:.2f} seconds at {fps} FPS).")
print(f"Output video saved to: {output_video_path}")
print(f"Log saved to: {log_path}")
MODEL_PATH = "Models/face_recognition2.pkl"
input_video = "test_files/aa.webm"
process_video(input_video,MODEL_PATH=MODEL_PATH)
Face Detection and Recognition on Live Stream
The code performs real-time face recognition using a webcam. It first loads pre-trained models—MTCNN for face detection and InceptionResnetV1 for feature extraction—from facenet_pytorch
, along with a previously trained SVM classifier and LabelEncoder
saved in face_recognition2.pkl
. The capture_face
function opens the webcam, runs for up to 10 seconds, and uses MTCNN to detect faces in real-time frames. Once a face is detected, it is cropped and displayed to the user. If no face is detected within the time limit, it exits gracefully. The captured face is then passed to recognize_face
, where the image is resized, normalized, and converted to a tensor before being embedded using InceptionResnetV1. This embedding is classified using the SVM, and if the prediction probability exceeds 0.6, the person’s name is printed; otherwise, the label “Unknown” is shown. The integration of webcam input and on-the-fly recognition allows for interactive and practical face identification applications.
Block Diagram
┌────────────────────────────┐
│ Load Pretrained Models │
│ - MTCNN for face detection │
│ - InceptionResnetV1 model │
└────────────┬───────────────┘
│
▼
┌────────────────────────────┐
│ Load Trained Classifier │
│ - SVM Classifier │
│ - LabelEncoder │
└────────────┬───────────────┘
│
▼
┌────────────────────────────┐
│ Activate Webcam │
│ Capture frames for 10 sec │
└────────────┬───────────────┘
│
▼
┌────────────────────────────┐
│ Detect Faces with MTCNN │
│ on each video frame │
└────────────┬───────────────┘
│
▼
┌──────────────────────────────────────────────┐
│ If a face is detected: │
│ - Crop and display face │
│ - Resize to 160x160 │
│ - Convert to tensor and normalize │
│ - Get embedding using InceptionResnetV1 │
│ - Predict identity using SVM │
│ - If prob > 0.6, label the person │
│ - Else label as "Unknown" │
└──────────────────────────────────────────────┘
│
▼
┌────────────────────────────┐
│ Display Final Prediction │
│ with Confidence Score │
└────────────────────────────┘
Code
import os
import torch
import numpy as np
import cv2
from facenet_pytorch import MTCNN, InceptionResnetV1
from sklearn.preprocessing import LabelEncoder
from sklearn.svm import SVC
import joblib
from IPython.display import display
from PIL import Image
import time
MODEL_PATH = "Models/face_recognition2.pkl"
# Load face detection and embedding models
device = torch.device("cuda" if torch.cuda.is_available() else "cpu")
mtcnn = MTCNN(image_size=160, margin=20, device=device)
resnet = InceptionResnetV1(pretrained="vggface2").eval().to(device)
# Load trained classifier
data = joblib.load(MODEL_PATH)
clf = data["classifier"]
encoder = data["encoder"]
def capture_face():
cap = cv2.VideoCapture(0) # Open webcam
if not cap.isOpened():
print("Error: Could not open webcam.")
return None
start_time = time.time()
captured_face = None
while time.time() - start_time < 10: # Run for 10 seconds
ret, frame = cap.read()
if not ret:
print("Failed to grab frame")
break
# Convert to RGB format for MTCNN
img_rgb = cv2.cvtColor(frame, cv2.COLOR_BGR2RGB)
img_pil = Image.fromarray(img_rgb)
# Detect faces
boxes, _ = mtcnn.detect(img_pil)
if boxes is not None:
for box in boxes:
x1, y1, x2, y2 = map(int, box)
# Crop the first detected face
captured_face = img_rgb[y1:y2, x1:x2]
# Show the detected face
display(Image.fromarray(captured_face))
cap.release()
cv2.destroyAllWindows()
return captured_face # Return the cropped face
cv2.imshow("Webcam - Detecting Face", frame)
if cv2.waitKey(1) & 0xFF == 27: # Press ESC to exit
break
cap.release()
cv2.destroyAllWindows()
print("No face detected within 10 seconds.")
return None
def recognize_face(face_image):
print("Hello")
if face_image is None:
print("No face to recognize!")
return
# Resize face for FaceNet
face = cv2.resize(face_image, (160, 160))
print("Face resized")
# Convert to tensor
face = torch.tensor(face).permute(2, 0, 1).float().unsqueeze(0) / 255.0
face = face.to(device)
# Extract embedding
embedding = resnet(face).detach().cpu().numpy().flatten()
# Predict identity
probs = clf.predict_proba([embedding])[0]
max_prob = max(probs)
predicted_label = encoder.inverse_transform([np.argmax(probs)])[0]
# Display result
person_name = predicted_label if max_prob > 0.6 else "Unknown"
print(f"Recognized Person: {person_name} ({max_prob:.2f})"
face = capture_face()
recognize_face(face)
Github link
The complete code of this tutorial is available at github.
Conclusion
We have successfully learned how to recognize a person’s face from an image, a video, or a live webcam stream. The process involved using MTCNN for face detection, InceptionResnetV1 to extract face embeddings, and an SVM classifier to identify the person based on those embeddings.